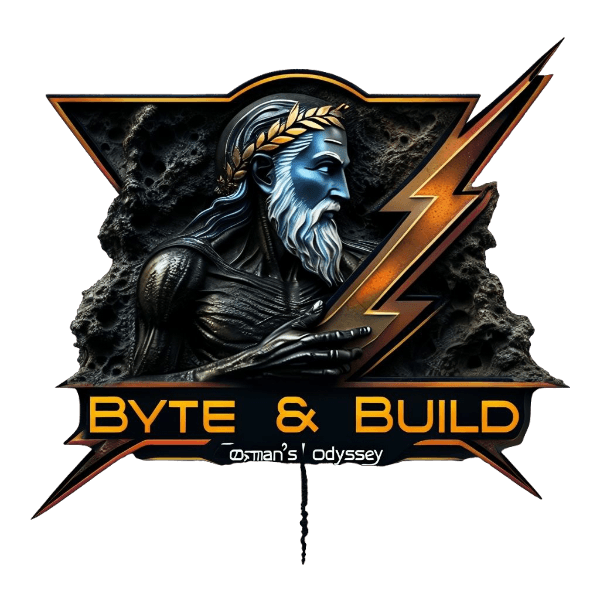
Blogs · · ·
-
Stop Wasting Multi-GPUs Setup—Use vLLM or ExLlamaV2 for Tensor Parallelism
Posted on
7 MinutesUse vLLM or ExLlamaV2 for Tensor Parallelism
Context: Yesterday, I watched @ThePrimeagen live stream (love his streams by the way) where he was stress testing his new Green Tinybox—a 6x RTX 4090 build. His plan was to get the LLM to send and receive concurrent messages and respond to each others, increasing the number and frequency of those messages with time, as a way to stress test those GPUs; and he was using llama.cpp for inference. The llama.cpp part got my attention, and with such a powerful setup, llama.cpp is pretty much a system crippler. Around the 26-minute mark of his stream, I commented on that, and after some back-and-forth, I figured it was best not to hijack his stream and just write this blogpost instead.
In one of his responses while live streaming, Michael(@ThePrimeagen) showed a GitHub thread about llama.cpp supporting concurrent requests, but that is more on the software threading side of things, and llama.cpp is not optimized for Tensor Parallelism and Batch Inference. In this blogpost, we dive into the details of various inference engines, explaining when each one makes sense depending on your setup. We’ll cover llama.cpp for CPU offloading when you don’t have enough GPU memory, how vLLM’s Tensor Parallelism gives a massive boost for multi-GPU systems with batch inference, and why ExLlamaV2’s EXL2 quantization is a great choice for Tensor Parallelism and Batch Inference when memory is limited, but not critically so.
In Short: an Inference Engine is a software that understands how to properly send human-input to, and in-turn show human-readable output from, these massive AI Models. In more detail, Large-Language Models (LLMs) are Deep Learning Neural Network Models. The LLMs we use right now come from an Architecture called Transformer which was coined in the infamous paper Attention Is All You Need . Inference Engines usually utilizes the Transformers implemented by the Hugging Face team, which on a lower-level supports the PyTorch, TensorFlow, and Jax libraries allowing for a wide variety of hardware support that those libraries provides tooling for.
The short version above is really all you need to know, so feel free to skip to the next section . But in case you’re curious, Inference Engines also implement a variety of other things that are not necessarily provided out of the box from the Transformers library, such as quantizations, and models’ architectures for those quantizations.
Are you still with me? Good. There are several layers to how an Inference Engine works. It starts with at the bottom level with the hardware you are running (CPU only, CPU and GPU Mixed, GPU only, TPU, NPU, etc), and then it looks into the details of that hardware (Intel, AMD ROCm, Nvidia Cuda, etc), then it goes one level higher and tries to figure out whether you are using a quantization (GGUF, exl2, AWQ, etc), or the original safetensor weights, and then the model architecture itself. The model architecture is the secret sauce—which sometimes is released in a training/white paper—of how the model does it magic to make meaningful connections of your input and then produce a—hopefully— meaningful output.
Imagine Large Language Models as complex Lego sets with Transformers as the basic bricks. However, each set has different instructions—some build from left to right, others can see the whole picture at once, and some might even shuffle the pieces around for a unique approach. Plus, each set might have its own special pieces or ways of snapping the Legos together, making each model unique in how it constructs or understands language.
There are many architectures out there, what we need to keep in mind is that:
This means code implementation for how they’re understood—AKA Inference—is also different.
llama.cpp is an Inference Engine that supports a wide-variety of models architectures and hardware platforms. It however does not support Batch Inference, making it less than ideal for more than one request at a time. It is mainly used with the GGUF quantization format, and the engines runs with an okay performance for single-request runs but not much else. The only time I would actually recommend using llama.cpp is when you do not have enough GPU Memory (VRAM) and need to offload some of the model weights to the CPU Memory (RAM).
-
Antifragile AI: Harnessing Uncertainty for a Resilient Future
Posted on
5 MinutesThe Evolution from Traditional Software to AI Agentic Systems
I came out of this experience with the following thesis: Given enough data, the aggregate of anyone’s thoughts—or anything’s properties and/or behaviors—could be simulated; a completely new paradigm to engaging with ideas and thoughts. The potential of contextual synthesis at scale is beyond our wildest imaginations, but I will leave exploring that to to future posts.
Building such a system wouldn’t be easy. It would require a significant amount of “bricolage” – piecing together various technologies and approaches in creative ways. But the potential rewards are immense.
Not long ago, I couldn’t grasp the urgency of ideas like Effective Accelerationism. Now, it feels like our only viable path forward. The rapid acceleration of AI capabilities demands we innovate, adapt, and build a future that uplifts everyone—no exceptions.
This isn’t just about keeping up; it’s about thriving in an era of unprecedented change. How do we stay antifragile in this relentless trajectory? By holding onto hope, pushing the boundaries of what’s possible, and shaping the future we want to see.
Now, let’s talk about Software.
Fundamentally, whether it’s a simple calculator or a sophisticated AI system, all computer programs can be thought of as agents that interact with their environment in some way. They perceive their environment through inputs and act upon it through their outputs and actions, even if their autonomy and adaptability vary widely.
The distinction between traditional software and the emerging paradigm of AI Agentic software is profound. This shift represents a fundamental change in how systems are designed, deployed, and interact with their environments, moving from robustness through iteration to antifragility, where systems not only withstand but thrive under uncertainty and stress.
Traditional software is characterized by its deterministic nature. It executes predefined algorithms and operates within a fixed framework, producing consistent outputs for given inputs. This approach is highly effective for tasks that are well-defined and require repeatability. However, this determinism also imposes limitations. Traditional software lacks the ability to adapt to new scenarios or learn from experience.
AI Agentic software, in contrast, embodies principles of adaptability and autonomy. These systems are designed to learn from their environment, make decisions based on available data, and adjust their behavior to achieve specified goals. They leverage machine learning algorithms, natural language processing, and other AI techniques to interpret complex inputs and generate contextually appropriate responses. In theory they can leverage anything and everything available to them, but we are not there yet!
-
All In — Stop Caring & Play The Game
Posted on
2 MinutesPlaying The Game Is The Only Way To Win
The truth is, it does not matter. I came to realize about a year ago that not a single thing I have achieved in life or done to completion was ever for a prize or a reward, and somehow these were the most fulfilling and the highest rewarding things. When I stopped caring and trusted my instincts, I won.
Life is a game. They always say that children’s curiosity is such an amazing thing, and in my opinion, it is more about them totally not caring about a single thing. Of course, that comes from a place of safety, as to having supportive and caring parents, nevertheless, the hypothesis holds.
With high stakes in life, you cannot always be risk-averse; however, when you have done your homework, and you know the underlying logic is valid thus the risk might be highly rewarding, you just have to override your brain’s defaults.
Life is a game. I like playing the game. I have always liked to play the game. I enjoy nothing in life than participating in it like a game. I am privileged to have been able to see it as a game. And the only way to honor that is by not letting my brain scare me away and to keep playing the game and winning.
-
Serving AI From The Basement — Part II: Agents, MoEs, Inference & More
Posted on
Last Edited — 14 MinutesUnpacking SWE Agentic Framework, MoEs, Batch Inference, and More
For about 3 weeks now, I have been working on a multi-agent system that simulates a team of Software Engineers; this system assigns projects, creates teams and adds members to them based on areas of expertise and need, and asks team members to build features, assign story points, have pair programming sessions together, etc. Started mainly for fun and exploration, however, last week the following paper was released: Agents in Software Engineering .
The paper delivers an overview of a framework that allows large language models play nicely within a sandbox for Software Engineering, and it cites several dozens of papers that implement task-specific agents. Since then, I have been a lot more motivated to get this agentic framework semi-decently put together, and it got me wondering: maybe it will beat Replit?
Overview of SWE Agentic Framework
Agents are Python scripts. Bash scripts. C++ programs. Or whatever. Agents are anything that can hit an OpenAI compatible API endpoint. Agents are anything that can talk with an inference engine sending inputs and receiving outputs. What makes them agentic is being permissive –while sandboxed– and having a few dozens of them running iterations for you to do A/B testing on.
I like playing with these toys because I do not know what the outcome might be. I really don’t. It differs from one model to another. Simple changes in Sampling Parameters might cause things to fully break or make things very interesting. It is a very fragile ecosystem right now.
However, I also believe that there is a very high possibility that what might not seem to be working with the current generation of models might be very feasible in a generation or two from now. So, I am going to build stupid toys, break them, iterate over them, and wait for a moment where something new, plug-and-play, becomes available for me throw in.
The time is 02:43 AM as I am writing this paragraph, Mr. Robot OST is playing in the background (all 8 volumes on loop, shuffled of course I am not an animal), and I just spent about ~5 hours on what I assumed would be a quick 2-3 mins task. In that time, I read about half a dozen quantization algorithms, another half dozen model architectures, and dove into GitHub exploring inference engines, libraries, and a lot of LLMOps tools that I was not aware of. I cannot sleep because I like when things work and I DO NOT like when things do not work. Stubbornness is essential when working in Software.
The vLLM inference engine, which I primarily use and is also widely utilized as a kernel in other engine implementations including SGlang , Aphrodite , and TensorRT-LLM , supposedly allows for Mixed Precision quantizations. However, the reality is more complex…
Well, as I said, it is complicated… My AI Server has 192GB of VRAM, and sometimes I would move my main RTX 4090 & RTX 3090 from my PC to the AI Server and that increases the VRAM to 240GB, and I am not typically a fan of that and neither is Tensor Parallelism. Llama 3.1 70B BF16 (Full Precision) has been my main driver model since release, and sometimes I switch to Llama 3.1 405B INT4 (Mixed Precision: 4-bits weights and 16-bits activation, aka W4A16).
-
Serving AI From The Basement — Part I: 192GB of VRAM Setup
Posted on
Last Edited — 3 MinutesA Dedicated AI Server with 8x RTX 3090 GPUs and 192GB of VRAM
This blogpost was originally posted on my LinkedIn profile in July 2024.
Backstory: Sometime in March I found myself struggling to keep up with the mere 48GB of VRAM I had been relying on for almost a year in my LLMs experimentations. So, in a geeky-yet-stylish way, I decided to spend my money to build this thing of beauty. Questions swirled: Which CPU/Platform to buy? Does memory speed really matter? And why the more PCIe Lanes we have the better? Why 2^n number of GPUs matter in multi-GPU node setup (Tensor Parallelism, anyone?) How many GPUs, and how can I get all the VRAM in the world? Why are Nvidia cards so expensive and why didn’t I invest in their stock earlier? What inference engine to use (hint: it’s not just llama.cpp and not always the most well-documented option)?
After so many hours of research, I decided on the following platform:
And we’re live!
Now that I kinda have everything in order, I’m working on a series of blog posts that will cover the entire journey, from building this behemoth to avoiding costly pitfalls. Topics will include:
Stay tuned.
P.S. I’m sitting here staring at those GPUs, and I just can’t help but think how wild tech progress has been. I remember being so excited to get a 60GB HDD back in 2004. I mean, all the movies and games I could store?! Fast forward 20 years, and now I’ve got more than triple that storage capacity in just one machine’s graphic cards… It makes me think, what will we be doing in another 20 years?!
Anyway, that’s why I’m doing this project. I wanna help create some of the cool stuff that’ll be around in the future. And who knows, maybe someone will look back on my work and be like “haha, remember when we thought 192GB of VRAM was a lot?”
Part II of this Blogpost Series is now available here.